Yates Continuity Correction Calculator
0.00
FAQs
How do you calculate Yates continuity correction? The Yates continuity correction is applied to a 2×2 contingency table in chi-square tests to correct for the potential overestimation of statistical significance. It involves adjusting the observed values in the table by subtracting 0.5 from the absolute difference between the observed and expected values in each cell. The formula for the corrected cell values is:
Corrected Cell Value = |Observed – Expected| – 0.5
You apply this correction to all four cells in the 2×2 table.
When should I use Yates continuity correction? Yates continuity correction is typically used when conducting a chi-square test with a 2×2 contingency table to account for the small sample size and the potential for inflated statistical significance. It is often applied in situations where expected cell counts are low (less than 5) and can help reduce the chance of Type I errors.
Is Fisher’s exact test better than Yates correction? Fisher’s exact test and Yates continuity correction serve different purposes. Fisher’s exact test is exact and does not rely on approximations like the chi-square test with Yates correction. It is often preferred when dealing with small sample sizes or when the assumptions of the chi-square test are not met. However, Fisher’s exact test can be computationally intensive for large datasets. The choice between them depends on the specific context and sample size.
What is a 2×2 contingency table? A 2×2 contingency table is a two-dimensional table used in statistics to summarize and analyze categorical data for two variables. It has two rows and two columns, making it a 2×2 matrix. Each cell in the table represents the frequency or count of specific combinations of values for the two variables, typically denoted as A and B.
What is the rule for continuity correction? The rule for continuity correction in a 2×2 contingency table is to adjust the observed values in each cell by subtracting 0.5 from the absolute difference between the observed and expected values. This correction helps prevent overestimation of statistical significance, especially when expected cell counts are small.
What is the Yates correction note? The Yates correction, named after the statistician Frank Yates, is a method used in the context of chi-square tests with 2×2 contingency tables to adjust for the discreteness of the data and potential overestimation of significance. It involves subtracting 0.5 from the absolute difference between observed and expected cell counts to achieve a more accurate test.
Should you always use the continuity correction? No, you should not always use the continuity correction. Whether to apply the Yates continuity correction depends on the specific situation and the characteristics of your data. It is typically used when dealing with small sample sizes or when expected cell counts are less than 5. For larger sample sizes and when assumptions are met, you may not need the continuity correction.
What is the difference between chi-square and Fisher’s exact test? Both chi-square and Fisher’s exact test are used for analyzing categorical data, but they differ in their assumptions and applications:
- Chi-square test: It is used to determine whether there is a significant association or independence between two categorical variables. It assumes that the expected cell counts are sufficiently large.
- Fisher’s exact test: It is used to test the independence of two categorical variables, particularly when dealing with small sample sizes or when the assumptions of the chi-square test are violated. Fisher’s exact test calculates the exact probability of obtaining the observed data under the null hypothesis.
Why not use Fisher’s exact test? While Fisher’s exact test is a powerful and exact method, it can be computationally intensive and may not be practical for large datasets. Additionally, when expected cell counts are not extremely low, the chi-square test with or without Yates continuity correction can provide reasonable approximations and is less computationally demanding.
Why not always use Fisher’s exact test? Fisher’s exact test may not always be the preferred choice because it can be computationally expensive and time-consuming for large datasets. Additionally, when expected cell counts are not extremely low, chi-square tests (with or without continuity correction) can provide reasonably accurate results and are computationally more efficient.
When should Fisher’s exact test be used? Fisher’s exact test should be used in situations where the assumptions of the chi-square test are violated, particularly when dealing with small sample sizes or when expected cell counts are very low (less than 5). It is a valuable tool when you need an exact test of independence.
What is the Yates continuity correction? The Yates continuity correction is a statistical adjustment applied to a 2×2 contingency table in chi-square tests to account for the discreteness of the data and prevent overestimation of statistical significance. It involves subtracting 0.5 from the absolute difference between the observed and expected values in each cell.
What does Fisher exact test tell you? Fisher’s exact test is used to determine whether there is a significant association or independence between two categorical variables in a contingency table. It calculates the exact probability of obtaining the observed data under the null hypothesis, allowing you to assess whether any observed associations are statistically significant.
What is Cramer’s V in chi-square? Cramer’s V is a measure of the strength of association between categorical variables in a chi-square analysis. It is used to quantify the effect size or the degree of relationship between the variables. It ranges from 0 to 1, with higher values indicating a stronger association.
Do you add or subtract 0.5 for continuity correction? You subtract 0.5 for continuity correction in a 2×2 contingency table. This adjustment is made to the observed values in each cell by subtracting 0.5 from the absolute difference between the observed and expected values.
What are the three rules of continuity? The three rules of continuity are guidelines for determining when to apply continuity correction in chi-square tests:
- Rule of 5: Apply continuity correction when any expected cell count is less than 5.
- Rule of 10: Avoid using chi-square tests altogether when more than 20% of the expected cell counts are less than 10.
- Rule of 1: If the sample size is small (less than 50), be cautious and consider using Fisher’s exact test instead.
What is the sum rule of continuity? The sum rule of continuity is not a commonly recognized term in statistics. It is possible that you meant to refer to one of the guidelines for applying continuity correction, such as the “Rule of 5” mentioned earlier.
Where is Yates correction used? Yates correction is primarily used in chi-square tests with 2×2 contingency tables. It is applied to adjust for the discreteness of the data and potential overestimation of statistical significance when dealing with small sample sizes or low expected cell counts.
What is the formula of correction factor? The correction factor formula for Yates continuity correction is:
Correction Factor = |Observed – Expected| – 0.5
This formula is applied to each cell in a 2×2 contingency table to adjust the observed values.
Why do you need to use the continuity correction when using the normal distribution to approximate the binomial distribution more accurately? The continuity correction is used when approximating the binomial distribution with the normal distribution to improve accuracy. Without the correction, the normal approximation can lead to inaccurate results, especially when dealing with small sample sizes or discrete data. By subtracting 0.5 from the observed values in a 2×2 contingency table, the continuity correction helps account for the discreteness of the data, resulting in a more accurate approximation when using the normal distribution.
What can I use instead of chi-square test? Instead of the chi-square test, you can consider using other statistical tests depending on your data and research question:
- Fisher’s Exact Test: For small sample sizes and 2×2 contingency tables when chi-square assumptions are not met.
- G-test: Similar to the chi-square test but more sensitive when expected cell counts are small.
- Likelihood Ratio Test: Used when comparing nested models and assessing the goodness-of-fit in contingency tables.
- Log-linear Models: Useful for analyzing multi-way contingency tables or when examining relationships between multiple categorical variables.
- McNemar’s Test: Specifically for paired nominal data.
The choice of test depends on the nature of your data and research objectives.
When would you use Fisher’s exact test instead of a test of independence? Fisher’s exact test is often preferred over a test of independence (such as the chi-square test) in the following situations:
- Small Sample Sizes: When dealing with small sample sizes where expected cell counts are low (typically less than 5).
- 2×2 Contingency Tables: Specifically, when working with 2×2 tables.
- Violation of Assumptions: When the assumptions of the chi-square test are not met (e.g., when expected cell counts are insufficiently large).
- Exact Testing: When you require an exact test of independence without relying on asymptotic approximations.
What assumptions are required for Fisher’s exact test? Fisher’s exact test does not have stringent assumptions like the chi-square test. However, it is typically used in situations where the following conditions apply:
- Categorical Data: The data should consist of categorical variables.
- Independence: The observations should be independent of each other.
- Fixed Margins: The row and column totals in the contingency table should be fixed in advance (i.e., no sampling without replacement).
Fisher’s exact test is robust and applicable in a wide range of scenarios, including situations where the assumptions of the chi-square test are not met.
What are the weaknesses of Fisher’s exact test? While Fisher’s exact test is a valuable tool, it has some weaknesses:
- Computational Complexity: It can be computationally intensive for large datasets, making it impractical in some cases.
- Sensitivity to Large Data: For very large datasets, the test may detect small, practically insignificant differences as statistically significant due to its exact nature.
- Focus on 2×2 Tables: Fisher’s exact test is most commonly used for 2×2 tables, and its utility diminishes for larger tables.
Does Fisher’s exact test give you a p-value? Yes, Fisher’s exact test provides a p-value. The p-value tells you the probability of observing the data or more extreme data under the null hypothesis of independence. A small p-value indicates evidence against the null hypothesis, suggesting a significant association between the variables in the contingency table.
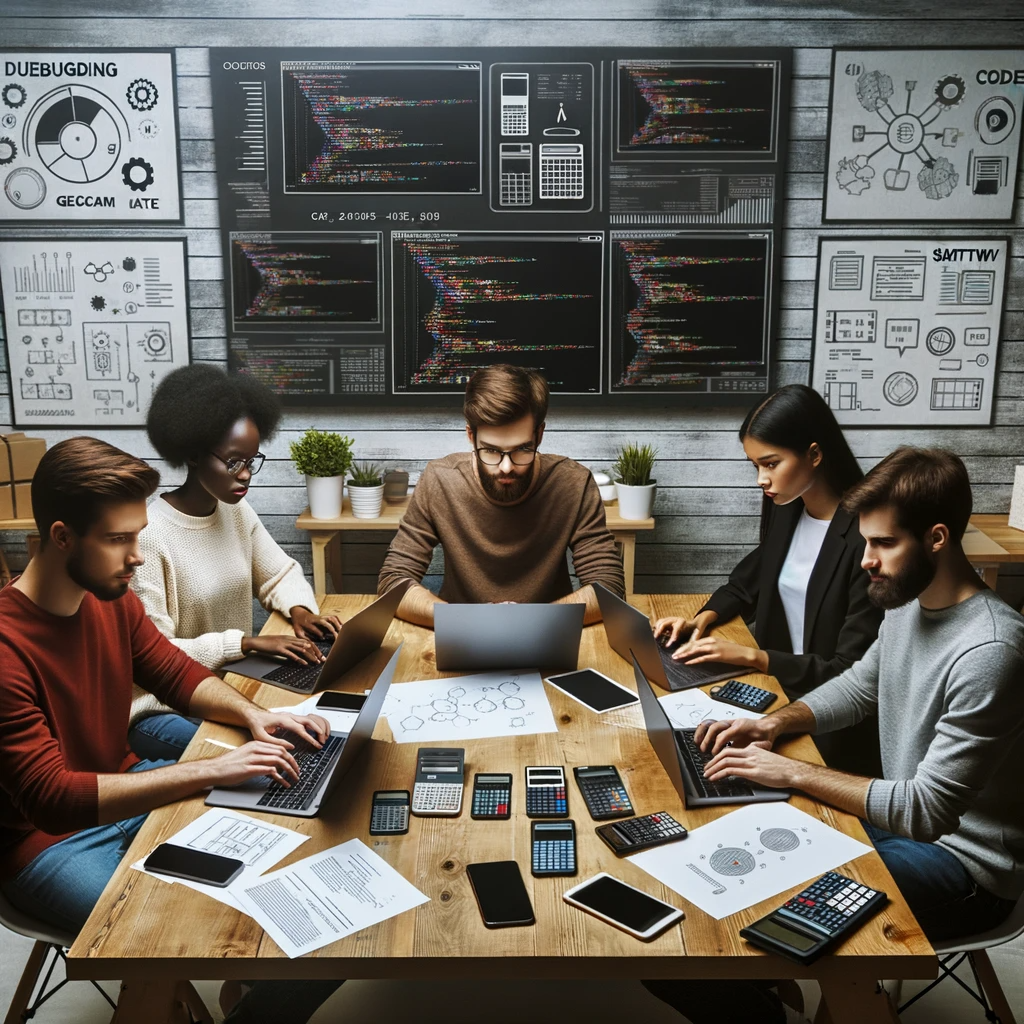
GEG Calculators is a comprehensive online platform that offers a wide range of calculators to cater to various needs. With over 300 calculators covering finance, health, science, mathematics, and more, GEG Calculators provides users with accurate and convenient tools for everyday calculations. The website’s user-friendly interface ensures easy navigation and accessibility, making it suitable for people from all walks of life. Whether it’s financial planning, health assessments, or educational purposes, GEG Calculators has a calculator to suit every requirement. With its reliable and up-to-date calculations, GEG Calculators has become a go-to resource for individuals, professionals, and students seeking quick and precise results for their calculations.