RNA-seq Power Calculator
Required sample size:
FAQs
How do you calculate 80% power sample size?
To calculate the sample size needed to achieve 80% power (assuming a two-tailed test at a 5% significance level), you typically use a statistical power formula. The formula depends on several factors, including the effect size, significance level, and variability of the data. An estimation could be:
Sample Size ≈ 64 / (Effect Size)^2
Where Effect Size is typically estimated based on prior knowledge or research. This formula provides an approximation and may vary depending on the specific statistical test and assumptions used.
How do you calculate sample size power?
Sample size and power are interrelated. To calculate sample size given a desired power level, you need to know the effect size, significance level, and variability. The formula is:
Sample Size = (Z_alpha + Z_beta)^2 * (Variance) / (Effect Size)^2
Where:
- Z_alpha is the critical value for your chosen significance level.
- Z_beta is the critical value for your desired power level.
- Variance is the estimated variance in your data.
- Effect Size is the size of the difference you want to detect.
How do you calculate study power?
Study power is calculated by determining the probability of correctly rejecting the null hypothesis when it is false. It depends on the significance level (alpha), effect size, and sample size. You can calculate it using statistical software or online calculators.
How much RNA is needed for RNA-seq?
The amount of RNA needed for RNA-seq depends on various factors, including the type of sample, sequencing technology, and library preparation method. As a rough estimation, you may need at least 100 nanograms (ng) to 1 microgram (μg) of high-quality total RNA for a standard RNA-seq library. However, specific requirements can vary, so it’s essential to consult the protocol provided by your chosen RNA-seq kit and platform.
What does 80% power mean?
An 80% power means that there is an 80% probability that your statistical test will correctly detect a true effect (if it exists) and reject the null hypothesis. In other words, if you were to conduct the same experiment multiple times, you would expect to obtain a statistically significant result 80% of the time when there is a real effect.
What is the sample size for 90% power?
The sample size for 90% power depends on various factors, including the effect size and significance level. However, as a rough estimation, you may need a larger sample size compared to 80% power. You can use a sample size calculator or statistical software to determine the specific sample size required for 90% power in your study.
What does a power of 0.8 mean?
A power of 0.8 (or 80%) means that there is an 80% probability that your statistical test will correctly detect a true effect if it exists. It indicates a relatively high likelihood of detecting a real effect, which is commonly considered acceptable in many scientific studies.
What does 90 power mean in statistics?
A power of 90% in statistics means that there is a 90% probability that your statistical test will correctly detect a true effect if it exists. It signifies a high probability of finding real effects in your study.
What is the difference between effect size and power?
Effect size refers to the magnitude or size of the difference or relationship you are trying to detect in a study. Power, on the other hand, measures the probability of detecting that effect if it truly exists. Effect size and power are related, as a larger effect size typically requires a smaller sample size to achieve a desired level of power.
What is sample size power?
Sample size power refers to the ability of a study to detect a true effect, given a specific sample size. It quantifies the likelihood of correctly rejecting the null hypothesis when it is false, based on the chosen sample size, significance level, and effect size.
Is P value and power the same?
No, p-value and power are not the same. A p-value measures the probability of obtaining the observed results (or more extreme results) if the null hypothesis is true. It indicates the evidence against the null hypothesis. Power, on the other hand, measures the probability of correctly rejecting the null hypothesis when it is false. It assesses the ability of a study to detect a true effect.
How do you know if a study has sufficient power?
A study is considered to have sufficient power if the calculated power is reasonably high, often at least 80% or higher. To determine if a study has sufficient power, you can use statistical power calculations before conducting the study. If the calculated power is below the desired threshold, you may need to increase the sample size or adjust other study parameters to achieve adequate power.
How many reads is enough for RNA-seq?
The number of reads required for RNA-seq depends on various factors, including the complexity of the transcriptome, sequencing depth, and research goals. In general, a common benchmark is to aim for at least 20-30 million reads per sample for standard RNA-seq experiments. However, more complex samples or specific research objectives may require higher read counts.
What is the minimum sample size for RNA-seq?
The minimum sample size for RNA-seq depends on your research goals and the statistical power you want to achieve. A typical minimum might be around 3-5 biological replicates per condition to ensure some degree of statistical reliability. However, larger sample sizes are often recommended to increase the power of detecting differential expression.
How much RNA for Illumina?
Illumina sequencing platforms are commonly used for RNA-seq. The amount of RNA required for Illumina sequencing depends on the specific library preparation protocol and the sequencing depth you desire. As a rough estimate, you may need 100 ng to 1 μg of high-quality total RNA for Illumina RNA-seq library preparation.
What is a good power value?
A good power value typically falls in the range of 80% to 90% or higher. However, the specific power value considered “good” can vary depending on the field of study and the accepted standards within that field. In many scientific disciplines, a power of 80% is often considered acceptable, while a power of 90% or higher is desirable.
Is 80% power acceptable?
An 80% power is generally considered acceptable in many scientific studies. It indicates a reasonable probability of detecting true effects. However, the acceptable level of power may vary depending on the specific research context and field.
Does increasing sample size increase power?
Yes, increasing the sample size typically increases the power of a study. A larger sample size provides more data points and reduces the standard error, making it easier to detect real effects. Increasing the sample size can enhance the statistical power to correctly reject the null hypothesis when it is false.
Is 200 a big enough sample size?
A sample size of 200 can be considered relatively large in many research contexts, but its adequacy depends on the specific research goals, effect size, and variability in the data. Whether 200 is “big enough” depends on the statistical power required to detect the effects of interest. Some studies may require larger sample sizes for sufficient power, while others may find 200 to be adequate.
Is 300 sample size enough?
A sample size of 300 is generally considered relatively large and should provide good statistical power for many types of research. However, the adequacy of a sample size depends on the specific research objectives, effect size, and variability in the data. It’s essential to perform power calculations to determine if 300 is sufficient for your study.
Is a sample size of 100 too small?
A sample size of 100 may be sufficient for some research studies, depending on the context and research goals. However, it may be considered small for certain types of analyses, particularly when detecting smaller effect sizes or dealing with high variability. To determine if a sample size of 100 is appropriate, it’s important to conduct power calculations and consider the specific objectives of your study.
What does 50% power mean in statistics?
A power of 50% means that there is a 50% probability of correctly detecting a true effect if it exists. In statistical terms, it represents a low probability of finding real effects and is generally considered inadequate for most research studies.
What does a power of 0.95 mean?
A power of 0.95 (or 95%) means that there is a 95% probability of correctly detecting a true effect if it exists. It signifies a high likelihood of finding real effects in your study and is often considered desirable in many scientific research contexts.
What is the power of 0.9 in statistics?
A power of 0.9 (or 90%) means that there is a 90% probability of correctly detecting a true effect if it exists. It indicates a high probability of finding real effects in your study and is commonly considered acceptable in many research fields.
What does a statistical power of 95% mean?
A statistical power of 95% means that there is a 95% probability of correctly detecting a true effect if it exists. It signifies a high likelihood of finding real effects in your study and is often considered a robust level of power.
Can statistical power be 100%?
In practice, it is exceedingly rare to achieve a statistical power of 100% because there is always some degree of uncertainty and variability in real-world data. However, researchers aim for high power values (e.g., 90% or 95%) to maximize their chances of detecting true effects.
How do you explain power in statistics?
In statistics, power is the probability that a statistical test will correctly detect a true effect (i.e., reject the null hypothesis) if that effect exists in the population being studied. It quantifies the ability of a study to find real relationships or differences when they are present. A higher power indicates a greater likelihood of detecting real effects, while lower power implies a higher risk of failing to detect true effects.
What is a good effect size?
A good effect size depends on the specific research context and goals. Effect sizes can vary widely across different fields of study. In some cases, a small effect size may be highly meaningful (e.g., in medical research), while in others, a large effect size may be required to have practical significance. What constitutes a good effect size is often determined by subject matter expertise and prior research in the field.
What does an effect size of 0.5 mean?
An effect size of 0.5 represents a moderate effect. It indicates that there is a meaningful and noticeable difference or relationship between groups or variables. The interpretation of effect sizes can vary depending on the context, but a value of 0.5 is often considered moderate and of practical significance.
How do you increase power in statistics?
To increase power in statistics, you can:
- Increase the sample size: A larger sample size reduces the standard error and increases the likelihood of detecting real effects.
- Adjust the significance level: Using a less stringent significance level (e.g., 0.10 instead of 0.05) can increase power but may increase the risk of Type I errors.
- Increase the effect size: Larger differences or stronger relationships are easier to detect.
- Reduce variability: Minimize sources of variability in your data.
- Choose appropriate statistical tests: Select tests that are more powerful for your specific research question.
What is the rule of 30 in statistics?
The “rule of 30” is not a standard statistical concept. It may refer to a general guideline that suggests having a minimum of 30 data points (or observations) for certain statistical analyses, such as parametric tests like t-tests or ANOVA. However, the adequacy of sample size depends on various factors, including the specific analysis, effect size, and variability, so it’s important to conduct power calculations and consult statistical guidelines for your particular study.
What is the relationship between effect size and power?
The relationship between effect size and power is inverse. A larger effect size (e.g., a more substantial difference or relationship between groups or variables) typically requires a smaller sample size to achieve a desired level of power. Conversely, a smaller effect size often necessitates a larger sample size to achieve the same level of power.
Does a smaller p-value mean more power?
No, a smaller p-value does not necessarily indicate more power. A smaller p-value indicates stronger evidence against the null hypothesis, but power measures the probability of correctly detecting a true effect if it exists. A study can have a small p-value but low power if the sample size is inadequate or if the effect size is small.
What is an example of a power of a test?
An example of a power of a test could be in a medical trial to test the effectiveness of a new drug. Let’s say the drug truly has a substantial effect in reducing symptoms. The power of the test in this context would represent the probability that the clinical trial correctly detects this effect (e.g., a reduction in symptoms) if it exists in the population of patients taking the drug.
Is power the same as probability?
Power in statistics is related to probability but is not the same thing. Power is the probability of correctly rejecting the null hypothesis when it is false (i.e., detecting a true effect). Probability, in a broader sense, refers to the likelihood of any event occurring, not necessarily related to hypothesis testing.
What three factors determine the power of a study?
The power of a study is determined by three main factors:
- Effect Size: The magnitude of the difference or relationship you want to detect in your study.
- Sample Size: The number of participants or data points in your study.
- Significance Level: The chosen level of significance (usually set at 0.05) that defines the probability of making a Type I error (incorrectly rejecting a true null hypothesis).
What is a statistically significant sample size?
A statistically significant sample size is one that is chosen to provide sufficient statistical power to detect meaningful effects or relationships in a study. It is determined through power calculations and is typically large enough to ensure that the study can achieve the desired level of power to detect real effects.
What is high power in a study?
High power in a study indicates a high probability of correctly detecting real effects or relationships if they exist. It signifies that the study is well-designed to minimize the risk of false negatives (Type II errors) and is more likely to yield reliable and robust results.
Should you trim your RNA-seq reads?
Trimming RNA-seq reads is a common preprocessing step to remove low-quality bases or adapter sequences. Trimming can improve the accuracy of downstream analysis and reduce noise. However, whether you should trim reads depends on the quality of your sequencing data and the specific requirements of your analysis. It’s generally advisable to assess the quality of your raw reads and, if needed, perform trimming as part of the data preprocessing pipeline.
What is the average read count for RNA-seq?
The average read count for RNA-seq experiments can vary widely depending on the study’s goals, sequencing depth, and the complexity of the transcriptome. However, for a standard RNA-seq experiment, an average of 20-30 million reads per sample is a common benchmark. Some studies may require higher read counts, while others with simpler transcriptomes may achieve meaningful results with fewer reads.
How many times can one mRNA be read?
In RNA-seq, one mRNA molecule can generate multiple reads during sequencing. The number of times an mRNA is read (sequenced) is determined by the sequencing depth, which is the total number of reads generated in the experiment. Sequencing depth can vary widely, but it’s common for each mRNA molecule to be represented by multiple reads to ensure accurate quantification and detect low-abundance transcripts.
How deep is enough in single-cell RNA-seq?
The required sequencing depth in single-cell RNA-seq (scRNA-seq) depends on the specific goals of the study and the complexity of the cell population. Generally, deeper sequencing with more reads per cell can provide greater sensitivity to detect rare transcripts and enhance the accuracy of gene expression quantification. A common guideline is to aim for 50,000 to 100,000 reads per cell for scRNA-seq experiments, but this can vary depending on the study’s objectives and budget constraints.
What is library size in RNA-seq?
Library size in RNA-seq refers to the total number of sequencing reads generated for a particular sample after library preparation and sequencing. It represents the raw count of reads that were obtained for that sample. Library size is a crucial factor in RNA-seq analysis, as it impacts downstream normalization and differential expression analysis.
How many cells do you need for bulk RNA-seq?
The number of cells required for bulk RNA-seq can vary depending on factors like the cell type, RNA quality, and sequencing depth. As a general guideline, you may need a few thousand to tens of thousands of cells for a typical bulk RNA-seq experiment. However, it’s important to consider the specific research goals and the complexity of the transcriptome when determining the appropriate number of cells.
What is the minimum amount of RNA?
The minimum amount of RNA required for various molecular biology experiments, including RNA-seq, can vary depending on the specific protocol and technology used. For standard RNA-seq library preparation, you may need at least 100 nanograms (ng) of high-quality total RNA. However, some protocols and platforms may require less RNA, while others may necessitate more. Always refer to the specific guidelines and requirements of your chosen RNA-seq kit and platform.
How do you quantify RNA amount?
RNA amount can be quantified using various methods, including:
- Spectrophotometry: UV absorbance at 260 nm is used to estimate RNA concentration.
- Fluorometry: Fluorescent dyes, such as RiboGreen, can bind to RNA and provide accurate quantification.
- Quantitative PCR (qPCR): RNA can be quantified by comparing its amplification to a standard curve of known RNA concentrations.
- Bioanalyzer or TapeStation: These instruments analyze RNA electrophoretically and provide concentration measurements.
- Nanodrop: Measures RNA concentration based on UV absorbance.
What is the lowest amount of RNA for cDNA?
The minimum amount of RNA required for cDNA synthesis depends on the specific reverse transcription (RT) or cDNA synthesis protocol and the downstream applications. Typically, a few nanograms (ng) of RNA is sufficient for most cDNA synthesis reactions. However, the exact requirement can vary, so it’s essential to follow the guidelines provided with your chosen cDNA synthesis kit.
Why use 80% power?
Using 80% power in statistical analyses is a common practice because it represents a reasonable balance between the ability to detect real effects and the practical constraints of sample size. An 80% power level is often considered acceptable in many scientific studies, as it provides a good chance of detecting meaningful effects while minimizing the need for extremely large sample sizes.
How to do power calculations for sample size?
To perform power calculations for sample size determination, you typically need to:
- Define your research goals and hypotheses.
- Determine the significance level (alpha) you’re willing to accept (e.g., 0.05).
- Choose a desired power level (e.g., 80% or 90%).
- Estimate the expected effect size and variability in your data.
- Use statistical software, calculators, or formulas to calculate the required sample size based on these parameters.
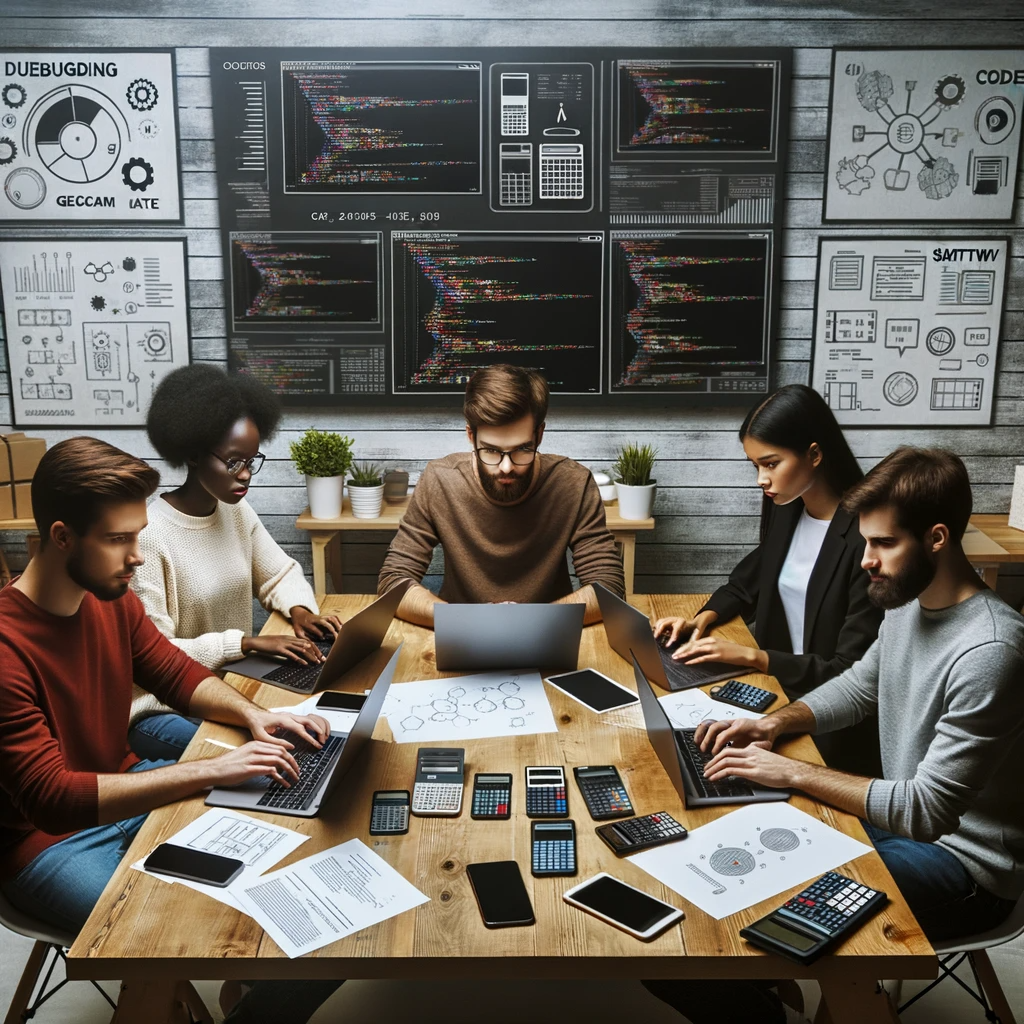
GEG Calculators is a comprehensive online platform that offers a wide range of calculators to cater to various needs. With over 300 calculators covering finance, health, science, mathematics, and more, GEG Calculators provides users with accurate and convenient tools for everyday calculations. The website’s user-friendly interface ensures easy navigation and accessibility, making it suitable for people from all walks of life. Whether it’s financial planning, health assessments, or educational purposes, GEG Calculators has a calculator to suit every requirement. With its reliable and up-to-date calculations, GEG Calculators has become a go-to resource for individuals, professionals, and students seeking quick and precise results for their calculations.