Post-Hoc Statistical Power Calculator for Multiple Regression
FAQs
How to do a post hoc power calculation? Post hoc power calculations involve estimating the statistical power of an already conducted study. To perform a post hoc power calculation, follow these steps:
- Gather data: Collect the sample size (n), effect size estimate (e.g., Cohen’s d for t-tests or odds ratio for logistic regression), and significance level (α) from your completed study.
- Calculate the observed test statistic: Use the collected data to calculate the test statistic (e.g., t-value, chi-square statistic) that was used in your original analysis.
- Choose a power analysis tool: You can use statistical software like G*Power or an online calculator for post hoc power analysis.
- Input the parameters: Enter the values you gathered into the power analysis tool, including the sample size, effect size, and significance level.
- Calculate post hoc power: The tool will provide you with the post hoc power estimate, which represents the likelihood of detecting an effect of the observed size at the given significance level with the sample size you used.
What is post hoc statistical power? Post hoc statistical power refers to the retrospective calculation of statistical power after a study has been conducted. It quantifies the ability of a study to detect an effect of a specific size (as indicated by the effect size used in the analysis) based on the sample size and significance level that were employed. It helps researchers assess whether their study had a sufficient sample size to detect the observed effect or whether the results may be inconclusive due to low power.
How to do a post hoc power analysis in SPSS? SPSS does not have a built-in feature for post hoc power analysis. You would typically need to use a dedicated statistical power analysis tool or software like G*Power or conduct the calculations manually using the collected data and appropriate formulas.
How to calculate sample size for multiple logistic regression? Calculating sample size for multiple logistic regression involves considering factors like the desired power, significance level, the number of predictors, and the expected effect size (odds ratio). You can use software or online calculators specifically designed for sample size calculation in logistic regression, as it can be complex. Alternatively, consult a statistician for assistance.
When to do a post hoc power analysis? You should consider conducting a post hoc power analysis when you have already completed a study and want to assess whether the sample size used in your study was adequate to detect the observed effects. This can be valuable in interpreting the validity and reliability of your study’s findings.
Is post hoc power analysis necessary? Post hoc power analysis is not always necessary, and its usefulness can be debated. It can provide insight into the adequacy of your sample size, but it cannot change the results of your completed study. It is often more valuable to conduct a priori power analysis before conducting a study to determine an appropriate sample size.
What does a statistical power of 95% mean? A statistical power of 95% means that if an effect truly exists in the population, your study has a 95% chance of detecting it as statistically significant (assuming all other factors are constant). In other words, it reflects a high probability of correctly rejecting the null hypothesis when it is false.
What does 50% power mean in statistics? A statistical power of 50% means that your study has a 50% chance of detecting an effect if it truly exists in the population. This represents a low probability of correctly rejecting the null hypothesis, indicating a high risk of failing to detect real effects.
Is Chi Square a post hoc? No, the chi-square test is not a post hoc test. The chi-square test is a statistical test used to assess the independence of categorical variables in a contingency table. Post hoc tests are typically used after conducting an analysis of variance (ANOVA) or a regression analysis to make specific comparisons between groups.
What is post hoc analysis in regression? Post hoc analysis in regression refers to additional analyses conducted after a regression model has been fit to the data. These analyses often involve examining specific pairwise comparisons between groups, variables, or levels to further understand the relationships uncovered by the regression model. Post hoc analyses can help identify which specific factors are driving the observed effects.
What is the p-value for post hoc analysis? In post hoc analysis, p-values are used to assess the statistical significance of specific comparisons or tests made after an initial analysis (e.g., ANOVA or regression). Each post hoc test generates its own p-value to determine whether the observed differences or relationships are statistically significant.
What is the difference between an a priori power analysis and post hoc power analysis? The main difference between these two types of power analysis is their timing:
- A priori power analysis is conducted before data collection and is used to determine the required sample size for a study to achieve a desired level of statistical power.
- Post hoc power analysis is conducted after data collection and assesses the actual power achieved based on the sample size, effect size, and significance level observed in the completed study.
What is a good sample size for multiple regression? A good sample size for multiple regression depends on several factors, including the number of predictors, the expected effect size, and the desired level of statistical power. As a rough estimation, a sample size of at least 100 participants is often considered a reasonable starting point for multiple regression. However, it is advisable to conduct a sample size calculation specific to your research context.
What is the power calculation for logistic regression analysis? The power calculation for logistic regression analysis is more complex than for simple tests. It involves factors such as the number of predictors, the expected effect size (odds ratio), the significance level, and the desired power. Using statistical software or calculators designed for logistic regression sample size calculation is recommended.
What effect size should I use for multiple regression? The effect size in multiple regression is typically represented by standardized regression coefficients (beta coefficients). The effect size you use depends on your research goals. If you want to assess the strength of the relationship between a predictor and the outcome, standardized coefficients are useful. Alternatively, you can use semi-partial correlations (part correlations) to gauge the unique contribution of each predictor.
How do you know if a post hoc test is significant? To determine if a post hoc test is significant, you would examine the p-value associated with the test. If the p-value is less than your chosen significance level (e.g., 0.05), you would consider the results of the post hoc test to be statistically significant, indicating that there are significant differences between the groups or conditions being compared.
What does a low post hoc power mean? A low post hoc power suggests that your study had a limited ability to detect the observed effects. It implies that there is a high risk of failing to find statistically significant results, even if there are true effects in the population. Low power can cast doubt on the reliability of your study’s conclusions.
How do you calculate post hoc analysis? Post hoc analysis involves conducting additional statistical tests or comparisons after an initial analysis (e.g., ANOVA, regression). The specific calculations depend on the type of test or comparison you want to perform. Typically, you would use appropriate statistical tests or procedures to compare groups or variables of interest, and the results would include p-values indicating statistical significance.
What is acceptable statistical power? Acceptable statistical power depends on the field of research and the specific study. In most research, a power of at least 80% is considered acceptable, but some fields or studies may require higher power levels. The choice of acceptable power should align with the goals of the research and the consequences of Type II errors (failing to detect a true effect).
When should post hoc not be used? Post hoc analyses should not be used as a substitute for proper experimental design and a priori power analysis. They are meant to explore unexpected findings or generate hypotheses rather than compensate for inadequate sample sizes or flawed study designs. It’s best to plan and conduct a study with an a priori power analysis to ensure adequate statistical power.
What is a good value for statistical power? A good value for statistical power depends on the specific research context and goals. As a general guideline, a power of 0.80 (80%) is often considered adequate in many fields. However, some studies may require higher power levels, especially when detecting small or important effects is crucial.
Is 80% statistical power enough? In many research contexts, 80% statistical power is considered acceptable. It means there’s an 80% chance of detecting an effect if it truly exists. However, the adequacy of 80% power depends on the specific research question, the consequences of Type II errors, and the field of study. Some studies may require higher power levels for more confidence in the results.
What does a statistical power of 0.8 mean? A statistical power of 0.8, or 80%, means that your study has an 80% chance of detecting a true effect if it exists. In other words, it reflects a high probability of correctly rejecting the null hypothesis when it is false.
What does a statistical power of 0.9 mean? A statistical power of 0.9, or 90%, means that your study has a 90% chance of detecting a true effect if it exists. It indicates a higher probability of correctly rejecting the null hypothesis compared to a lower power level.
Can statistical power be 100%? Statistical power is theoretically limited to a maximum of 100%. However, achieving 100% power is rare in practice and may require an infinitely large sample size, which is usually not feasible. In most research settings, researchers aim for a practical and reasonable level of power, often in the range of 80% to 95%.
What is the difference between P value and power? The main differences between p-value and power are:
- P-value: It indicates the probability of obtaining observed results (or more extreme) if the null hypothesis is true. A smaller p-value suggests stronger evidence against the null hypothesis.
- Power: It indicates the probability of correctly rejecting the null hypothesis when it is false. Higher power indicates a greater ability to detect true effects.
In summary, p-value assesses the evidence against the null hypothesis, while power assesses the ability to detect effects when they exist.
How do I know if a study is underpowered? A study is considered underpowered if it has a low statistical power, typically below 80%. You can determine if a study is underpowered by conducting a post hoc power analysis after data collection. If the calculated power is low, it suggests that the study may not have had sufficient sample size to detect the observed effects reliably.
Which post hoc should I use? The choice of which post hoc test to use depends on the type of statistical analysis you have conducted (e.g., ANOVA, regression) and the specific comparisons you want to make. Common post hoc tests include Tukey’s HSD, Bonferroni correction, Scheffé’s method, and more. Consult statistical guidelines and consider the nature of your research question to select the most appropriate post hoc test.
Which post hoc to use for ANOVA? For analysis of variance (ANOVA), the choice of post hoc test depends on whether the assumption of equal variances (homoscedasticity) is met and the specific comparisons you want to make. Common post hoc tests for ANOVA include:
- Tukey’s Honestly Significant Difference (HSD): Suitable when variances are equal.
- Bonferroni correction: Can be used when variances are not necessarily equal.
- Scheffé’s method: Appropriate for unequal variances and complex designs.
- Dunnett’s test: Used when comparing groups to a control group.
The choice should align with your data and research question.
What is post hoc ANOVA? Post hoc ANOVA, often referred to as post hoc analysis of variance, involves conducting additional tests or comparisons after an initial ANOVA to determine which group means are significantly different from each other. These tests help identify specific group differences when the ANOVA indicates a significant overall effect.
Should I use Bonferroni or Tukey? The choice between Bonferroni and Tukey’s HSD (Honestly Significant Difference) post hoc tests depends on your specific research context and goals:
- Bonferroni correction: It is more conservative and suitable when you want to control the familywise error rate (reduce the chance of Type I errors) when conducting multiple comparisons. It is a good choice when you have a small sample size or when you want to be cautious about false positives.
- Tukey’s HSD: It is less conservative and maintains a balance between Type I and Type II errors. It is appropriate when you have a larger sample size and are primarily interested in identifying which specific group means are significantly different from each other.
Choose the test that aligns with your research objectives and statistical considerations.
Is Tukey or Bonferroni post hoc test better? Neither Tukey’s HSD nor the Bonferroni correction is inherently “better” than the other. The choice between them depends on your specific research context and goals.
- Tukey’s HSD is less conservative and better at detecting true differences between group means when they exist. It’s often a good choice when you have a larger sample size and are primarily interested in identifying specific group differences.
- Bonferroni correction is more conservative and controls the familywise error rate, reducing the chance of Type I errors. It’s useful when you want to be cautious about making false positive claims, especially with smaller sample sizes.
The choice should align with your research objectives and the trade-off between Type I and Type II errors.
What is Tukey’s post hoc test? Tukey’s post hoc test, also known as Tukey’s Honestly Significant Difference (HSD), is a statistical method used after conducting an analysis of variance (ANOVA) to determine which group means are significantly different from each other. It is designed to maintain an appropriate balance between Type I and Type II errors and is particularly useful when you have a larger sample size.
What is a post-hoc analysis Bonferroni? A post-hoc analysis with Bonferroni correction involves conducting multiple pairwise comparisons after an initial analysis (e.g., ANOVA) and adjusting the significance level for each comparison to control the familywise error rate. The Bonferroni correction is a conservative method that reduces the risk of Type I errors when conducting multiple comparisons.
What is the p-value in Bonferroni? In Bonferroni correction, the p-value for each pairwise comparison is adjusted to control the familywise error rate. The adjusted p-value is typically denoted as “p-adjusted” or “p-Bonferroni.” It represents the significance level required for each individual comparison to maintain an overall significance level that controls for multiple comparisons.
What is the p-value in Tukey? In Tukey’s Honestly Significant Difference (HSD) post hoc test, the p-value for each pairwise comparison represents the probability of observing the observed differences in group means (or more extreme differences) if there were no true differences in the population. A smaller p-value indicates that the observed difference is statistically significant.
What is the formula for calculating statistical power? The formula for calculating statistical power depends on the specific statistical test being used. In general, statistical power is calculated using the following formula:
Power = 1 – β
Where:
- Power (1 – β) represents the probability of correctly rejecting the null hypothesis when it is false.
- β (beta) represents the probability of Type II error (failing to reject the null hypothesis when it is false).
The specific calculation can vary for different statistical tests and analyses.
How to do power calculations for sample size? To perform power calculations for sample size, you typically need to specify the following parameters:
- Effect size (e.g., Cohen’s d, odds ratio, R-squared).
- Significance level (α), often set at 0.05.
- Desired power (1 – β), typically set at 0.80 or higher.
- Type of statistical test (e.g., t-test, ANOVA, regression).
You can use statistical software like G*Power or online calculators to input these parameters and determine the required sample size to achieve the desired power.
Is G power the same as power analysis? GPower is a software tool commonly used for conducting power analysis, sample size calculations, and related statistical computations. So, in practice, GPower is often used for power analysis and sample size determination.
What is a good R-squared for multiple regression? A “good” R-squared value for multiple regression depends on the field of study and the research context. However, as a general guideline:
- A low R-squared (e.g., below 0.30) suggests that the predictors explain a small proportion of the variance in the dependent variable.
- A moderate R-squared (e.g., between 0.30 and 0.70) suggests a reasonable degree of explanatory power.
- A high R-squared (e.g., above 0.70) indicates that a substantial proportion of the variance in the dependent variable is explained by the predictors.
The appropriateness of R-squared also depends on the specific goals and complexity of the research.
What is the sample size required in a multiple regression with 5 independent variables? The sample size required for a multiple regression analysis with 5 independent variables depends on several factors, including the desired power, significance level, expected effect sizes of the predictors, and the presence of additional variables (e.g., control variables). As a rough estimation, you might need a sample size of at least 100 to 150 participants for a multiple regression with 5 independent variables. However, conducting a sample size calculation specific to your study is advisable.
How do you know if a multiple regression model is good? To assess the goodness of fit for a multiple regression model, you can consider several factors:
- R-squared (R^2): A higher R-squared indicates that a larger proportion of the variance in the dependent variable is explained by the independent variables. However, R-squared should be interpreted in the context of your research goals and field.
- Adjusted R-squared: Adjusted R-squared accounts for the number of predictors in the model and is useful for models with multiple independent variables.
- Residual Analysis: Examine the residuals (errors) to ensure they meet the assumptions of linear regression, such as normality and homoscedasticity.
- Significance of Independent Variables: Assess the p-values associated with each independent variable to determine whether they significantly contribute to the model.
- Effect Size: Consider the effect sizes (e.g., beta coefficients) to understand the practical importance of each predictor’s contribution.
- Cross-Validation: Use techniques like cross-validation to assess the model’s predictive performance on new data.
- Subject Matter Expertise: Consult with experts in your field to evaluate the model’s theoretical and practical relevance.
What is a good power for regression? A good power for regression analysis depends on the specific research context and goals. In many cases, a power of at least 80% is considered acceptable. However, the power you aim for should be determined by factors like the importance of the research question, the consequences of Type II errors, and the available resources.
What is the rule of thumb for logistic regression sample size? A common rule of thumb for logistic regression is to have a minimum of 10-20 events (cases where the outcome occurs) for each predictor variable. This guideline helps ensure that you have a sufficient sample size to estimate the parameters reliably. However, the adequacy of sample size can vary depending on the specific context and research goals, and it’s advisable to consult statistical guidelines or experts.
How to interpret multiple logistic regression? Interpreting multiple logistic regression involves considering the coefficients (log-odds or odds ratios) associated with each predictor variable and their p-values. Key steps in interpretation include:
- Coefficient Sign: Determine whether the coefficient for each predictor is positive or negative. A positive coefficient indicates that an increase in the predictor is associated with an increase in the log-odds (or odds) of the outcome, while a negative coefficient indicates the opposite.
- Coefficient Magnitude: Examine the magnitude of the coefficients. Larger coefficients suggest a stronger association with the outcome.
- P-Values: Assess the p-values associated with each coefficient. A low p-value (typically below 0.05) suggests that the predictor is statistically significant in predicting the outcome.
- Odds Ratios: If using odds ratios, interpret them as multiplicative changes in the odds of the outcome for a one-unit change in the predictor, while holding other predictors constant.
- Model Fit: Consider the overall fit of the model, such as the Hosmer-Lemeshow goodness-of-fit test and the Nagelkerke R-squared.
- Confidence Intervals: Examine the confidence intervals for coefficients to understand the precision of the estimates.
- Domain Knowledge: Incorporate domain-specific knowledge to interpret the practical significance of the results.
What is the R-squared effect size for multiple regression? In multiple regression, R-squared (or adjusted R-squared) represents the proportion of variance in the dependent variable that is explained by the independent variables included in the model. It is a measure of the model’s goodness of fit. R-squared values range from 0 to 1, with higher values indicating a better fit and more explanatory power.
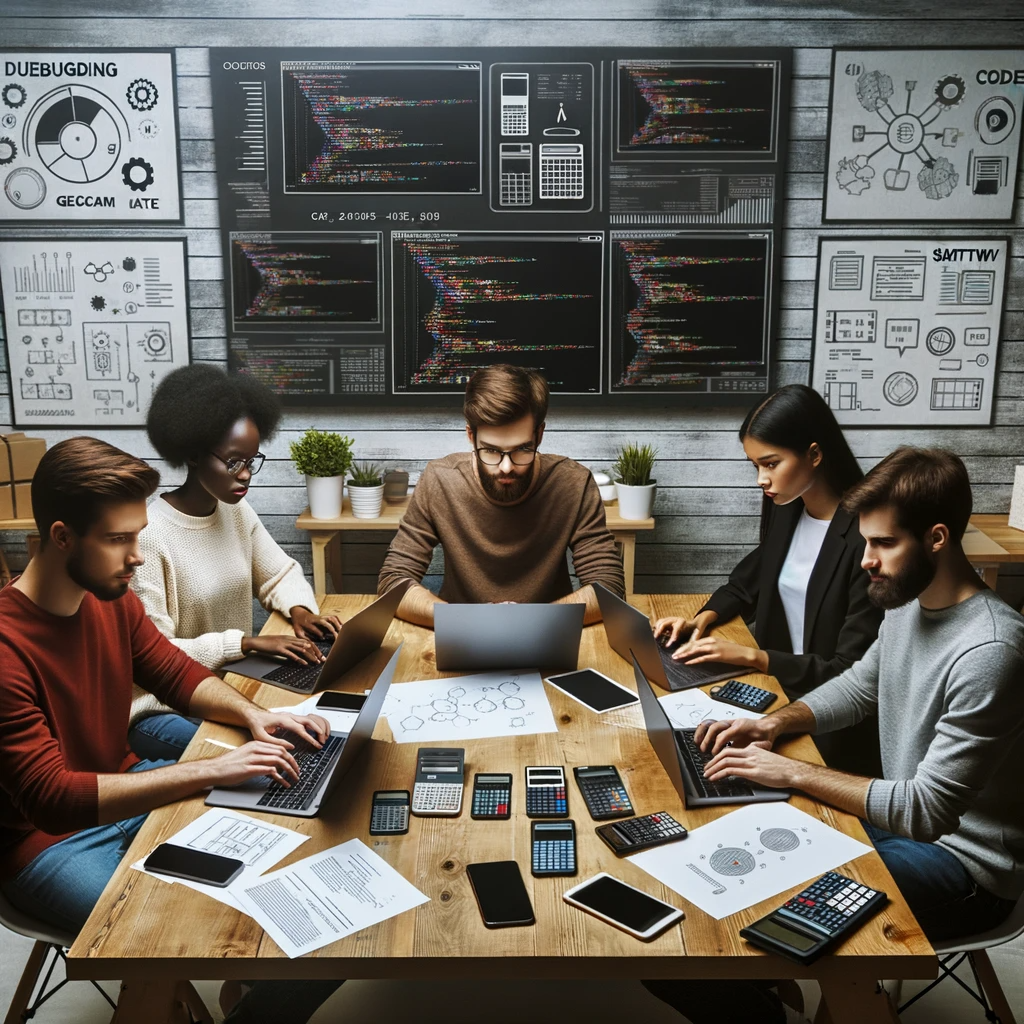
GEG Calculators is a comprehensive online platform that offers a wide range of calculators to cater to various needs. With over 300 calculators covering finance, health, science, mathematics, and more, GEG Calculators provides users with accurate and convenient tools for everyday calculations. The website’s user-friendly interface ensures easy navigation and accessibility, making it suitable for people from all walks of life. Whether it’s financial planning, health assessments, or educational purposes, GEG Calculators has a calculator to suit every requirement. With its reliable and up-to-date calculations, GEG Calculators has become a go-to resource for individuals, professionals, and students seeking quick and precise results for their calculations.