Minimum Detectable Effect Size Calculator
FAQs
How do you find the minimum detectable effect? The minimum detectable effect (MDE) is typically determined based on factors such as the desired level of significance (alpha), statistical power (1-beta), baseline or control group conversion rate, and expected or desired conversion rate in the treatment group. You can use statistical calculators or software to estimate the MDE for a given experiment or A/B test.
What is the minimal detectable effect size (MDE)? The minimal detectable effect size (MDE) is the smallest difference between groups or conditions that can be detected as statistically significant with a given level of confidence and power in a research study or experiment.
What is the difference between sample size and MDE? Sample size refers to the number of individuals or data points in a study, while MDE (minimal detectable effect size) is the smallest effect size that can be detected with a specified sample size, significance level, and power.
What is the minimum effect size required for statistical significance? The minimum effect size required for statistical significance depends on the specific statistical test being used, the chosen level of significance (alpha), and the sample size. A smaller effect size may require a larger sample size to achieve statistical significance.
What is the formula for effect size? The formula for effect size varies depending on the statistical test being used. Common effect size measures include Cohen’s d for t-tests, eta-squared (η²) for ANOVA, and Pearson’s r for correlation. Each has its own formula for calculation.
What is minimum detected value? “Minimum detected value” is not a standard statistical term. It may refer to the smallest value that can be detected or measured in a dataset, but it is not a commonly used term in statistics.
How do you calculate effect size using MCID? To calculate effect size using the Minimum Clinically Important Difference (MCID), you would typically compare the difference between two groups (e.g., treatment and control) in a clinical or medical context to determine if the observed effect is clinically meaningful. The specific calculation can vary depending on the context and measurement used.
What does MDE mean in statistics? MDE stands for “Minimum Detectable Effect” in statistics. It represents the smallest effect size that can be detected as statistically significant with a given level of confidence and power in a research study or experiment.
What is the MDE in hypothesis testing? In hypothesis testing, the MDE (Minimum Detectable Effect) is the smallest difference or effect size that an experiment or study can reliably detect as statistically significant. It is used to determine the required sample size to achieve a desired level of power and significance.
What is MDE in data? MDE in the context of data typically refers to the Minimum Detectable Effect, which is a statistical concept related to the smallest effect size that can be reliably detected in a data analysis.
What is the minimum effect size? The minimum effect size is the smallest meaningful or practical difference or effect that researchers or analysts are interested in detecting in a study. It is often defined based on the context of the research or the goals of the experiment.
What is an acceptable effect size? Acceptable effect size varies depending on the field of study and research objectives. In some cases, even a small effect size may be considered acceptable if it has practical significance. Researchers often determine what effect size is acceptable based on the specific context and goals of their study.
Do I report effect size if not significant? Yes, it is generally good practice to report effect size even if the results are not statistically significant. Effect size provides valuable information about the magnitude of an observed effect, regardless of whether it reaches statistical significance. This can help readers assess the practical importance of the findings.
How do you calculate the effect size difference? The calculation of effect size difference depends on the specific statistical test being used. For example, in a t-test, Cohen’s d is a commonly used measure of effect size difference, calculated as the difference between group means divided by the pooled standard deviation.
What does effect size tell you? Effect size tells you the magnitude of the difference or relationship between two groups or variables in a study. It provides a standardized measure of the practical significance of an observed effect, helping researchers and readers assess the real-world importance of the findings.
Is Cohen’s d the same as effect size? Cohen’s d is one of several measures of effect size used in statistics, particularly for comparing means between groups. Effect size is a broader term that encompasses various measures, including Cohen’s d, eta-squared (η²), and others, depending on the statistical test and context.
What is the minimum detectable change in statistics? The minimum detectable change (MDC) in statistics refers to the smallest change in a measurement or variable that can be reliably detected with a given level of confidence and statistical power. It is often used in clinical and scientific assessments.
What is minimum detectable difference stats? Minimum detectable difference (MDD) in statistics is similar to the minimum detectable change (MDC) and represents the smallest difference or change in a measurement or variable that can be detected with a specified level of confidence and power.
What is the minimum detectable change in reliability? The minimum detectable change in reliability (MDC-R) is a statistical measure used to assess the minimum change in a reliability coefficient (e.g., Cronbach’s alpha) that can be detected as statistically significant with a given level of confidence and power.
What is the minimum detectable difference and power? The minimum detectable difference (MDD) and power are related in that they both depend on factors such as sample size, effect size, and level of significance. Increasing power typically requires a larger sample size, which can also affect the minimum detectable difference.
How do you find the mean median and MDE? To find the mean and median of a dataset, you perform standard calculations based on the dataset’s values. The Minimum Detectable Effect (MDE) is not directly related to finding the mean or median and typically involves calculations related to statistical hypothesis testing and experimental design.
What is the minimum effect of interest? The minimum effect of interest (MOI) is the smallest effect size or difference that is considered practically or scientifically significant in a research study. It is often defined based on the context and goals of the study.
What is the difference between relative and absolute MDE? Relative MDE considers the effect size as a proportion or percentage of the baseline value, while absolute MDE considers the effect size as an absolute difference. For example, if you have a baseline conversion rate of 10% and a relative MDE of 20%, the MDE would be 12% (10% + 20% of 10%). In contrast, the absolute MDE would be 2% (10% + 20%).
What are the three methods used to test hypotheses in statistics? The three commonly used methods to test hypotheses in statistics are:
- Null Hypothesis Testing: Comparing observed data to a null hypothesis to determine if there is a significant difference.
- Bayesian Inference: Using Bayesian methods to update prior beliefs based on new data.
- Confidence Intervals: Estimating a range of values for a parameter to assess uncertainty.
What is MDI and MDE? MDI may refer to “Minimum Detectable Intensity” in some contexts and is related to the smallest intensity or magnitude that can be detected in measurements. MDE, as previously discussed, stands for “Minimum Detectable Effect” and relates to the smallest effect size detectable in statistical analysis.
What is the relationship between power and MDE? Power and MDE are inversely related. As you increase the desired level of power (i.e., the probability of detecting a true effect), you often need to increase the sample size, which can reduce the MDE (i.e., make it easier to detect smaller effects).
What is Alpha in MDE? Alpha (�α) in the context of MDE represents the level of significance chosen for a statistical test. It is typically set as the probability of Type I error (rejecting the null hypothesis when it is true) and is used to calculate the critical values for hypothesis testing.
What is effect size for dummies? “Effect size for dummies” is a colloquial term referring to a simplified explanation or introduction to the concept of effect size in statistics. Effect size measures the practical significance or magnitude of an effect in research or data analysis.
What is an example of effect size? An example of an effect size could be Cohen’s d, which measures the standardized difference between two group means. For instance, if a treatment group has a mean score that is 0.50 standard deviations higher than a control group, Cohen’s d would be 0.50.
How do you report effect size? Effect size is typically reported along with its corresponding measure (e.g., Cohen’s d, eta-squared, Pearson’s r). For example: “The effect size (Cohen’s d) for this experiment was 0.75, indicating a moderate effect.”
What is the rule of thumb for effect size? A common rule of thumb for interpreting effect sizes, particularly for Cohen’s d, is:
- Small effect: d = 0.20
- Medium effect: d = 0.50
- Large effect: d = 0.80
These values provide a general guideline for assessing the magnitude of effect sizes.
What is the benchmark for effect size? There is no universally fixed benchmark for effect size. The benchmark or threshold for what is considered a meaningful effect size depends on the field of study, research goals, and context.
Is 0.3 a good effect size? A Cohen’s d of 0.3 is generally considered a small to moderate effect size, depending on the context. Whether it is considered “good” depends on the specific research goals and the field of study.
How do you interpret not significant results? Interpreting nonsignificant results means that there is not enough evidence to reject the null hypothesis. It does not necessarily mean that there is no effect; it may be due to factors like sample size or variability. Researchers should carefully consider the practical implications of nonsignificant results.
Can you have a statistically significant result and have a small effect size? Yes, it is possible to have a statistically significant result with a small effect size. Statistically significant results mean that there is evidence of an effect, but the effect size indicates the magnitude of that effect, which may be small or practically insignificant.
What effect size do we report for correlation? For correlation, the effect size is typically reported as Pearson’s r, which measures the strength and direction of the linear relationship between two variables. The value of r ranges from -1 to 1, with 0 indicating no linear relationship.
What are the Cohen’s guidelines? Cohen’s guidelines provide a common framework for interpreting Cohen’s d effect sizes:
- Small effect: d = 0.20
- Medium effect: d = 0.50
- Large effect: d = 0.80
These guidelines help assess the practical significance of observed effects.
How do you interpret Cohen’s d effect size? Cohen’s d effect size can be interpreted as follows:
- Small effect: d ≈ 0.2 – The effect is small and may have limited practical significance.
- Medium effect: d ≈ 0.5 – The effect is moderate and has practical relevance.
- Large effect: d ≈ 0.8 – The effect is large and has substantial practical significance.
What are the three ways the effect size is usually measured? The three common ways effect size is usually measured are:
- Cohen’s d: Standardized difference between means.
- Eta-squared (η²): Proportion of variance explained in ANOVA.
- Pearson’s r: Correlation coefficient measuring the strength of a linear relationship.
How do you report the effect size of an independent t-test? To report the effect size of an independent t-test, you typically include Cohen’s d. For example: “The independent t-test revealed a significant difference between groups (t = 2.45, df = 48, p < 0.05, Cohen’s d = 0.50, indicating a moderate effect).”
What does an effect size of 0.4 mean? An effect size of 0.4, typically interpreted as Cohen’s d, indicates a moderate effect. It suggests that there is a meaningful and practical difference or relationship, but it may not be large.
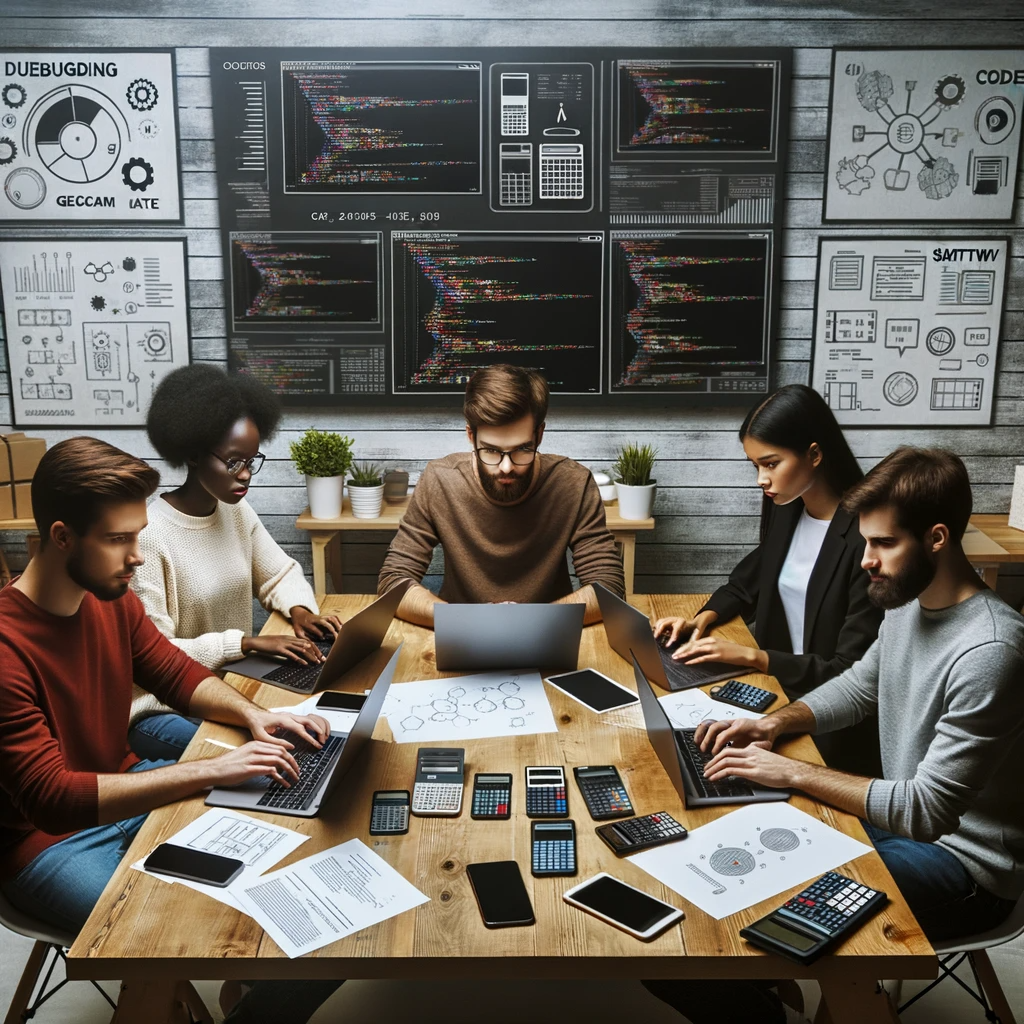
GEG Calculators is a comprehensive online platform that offers a wide range of calculators to cater to various needs. With over 300 calculators covering finance, health, science, mathematics, and more, GEG Calculators provides users with accurate and convenient tools for everyday calculations. The website’s user-friendly interface ensures easy navigation and accessibility, making it suitable for people from all walks of life. Whether it’s financial planning, health assessments, or educational purposes, GEG Calculators has a calculator to suit every requirement. With its reliable and up-to-date calculations, GEG Calculators has become a go-to resource for individuals, professionals, and students seeking quick and precise results for their calculations.